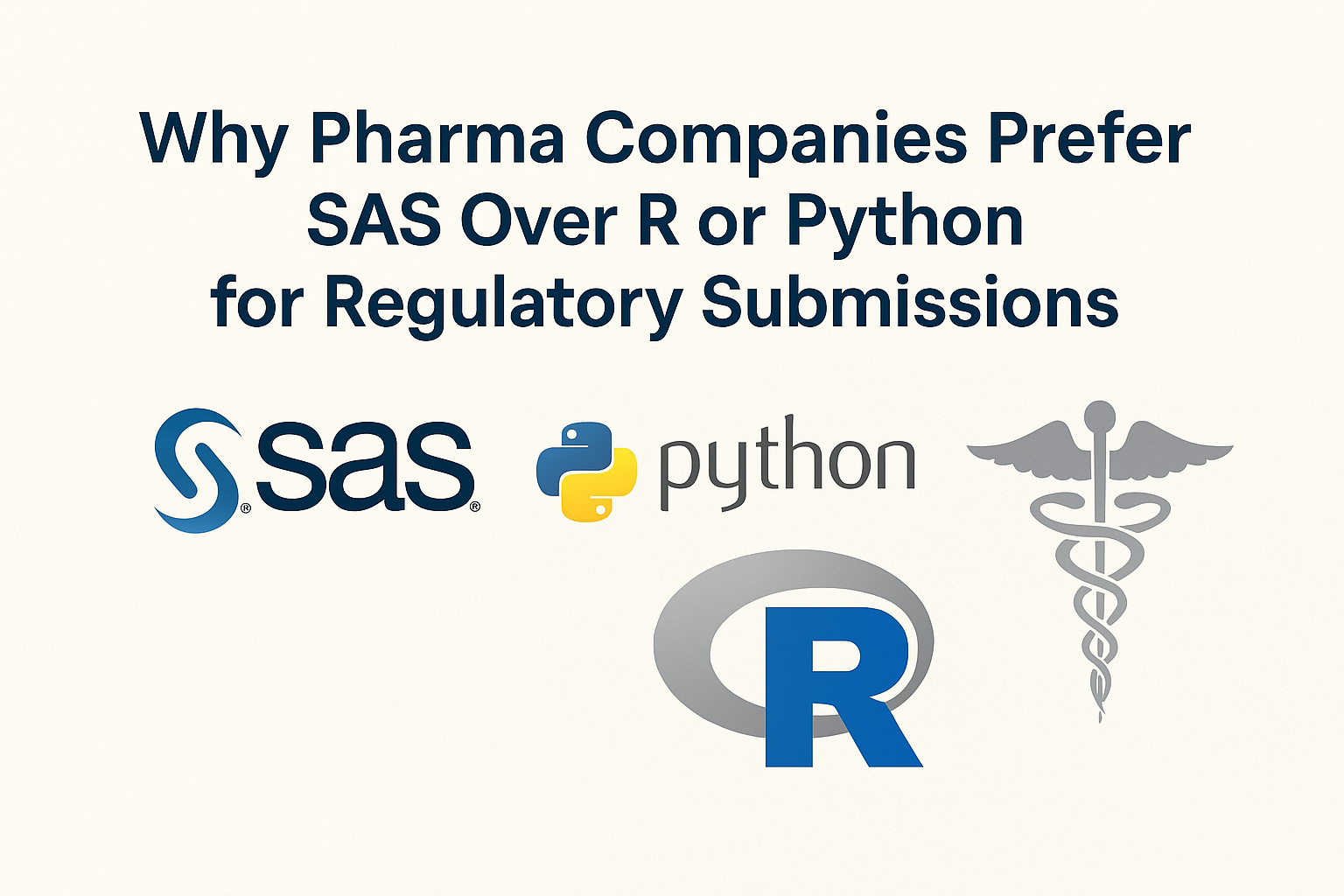
- by Handson
- April 8, 2025
Why Pharma Companies Prefer SAS Over R or Python for Regulatory Submissions
Why Pharma Companies Prefer SAS Over R or Python for Regulatory Submissions
In the highly regulated world of drug development, where patient safety and compliance are paramount, pharmaceutical companies and CROs must rely on tools that are not just powerful but also trusted by global regulators. While R and Python are widely used in data science, SAS (Statistical Analysis System) continues to be the preferred choice for clinical trial data analysis and regulatory submissions.
Here’s why SAS still leads the pack in life sciences and clinical research.
1. Regulatory Trust and Industry Acceptance
Regulatory agencies like the FDA, EMA, and PMDA have decades of experience working with SAS. In fact, many of these agencies use SAS themselves to review and validate sponsor-submitted data. This familiarity reduces risk and ensures faster review cycles.
SAS has built a reputation for robustness and auditability, which is exactly what regulators expect from tools used in clinical data submission. If you're preparing for a career in this domain, learning SAS gives you a critical edge in the job market. Learn how to start your Clinical SAS journey.
2. Built-In CDISC Support
SAS simplifies compliance with essential data standards like CDISC, SDTM, and ADaM. Its toolkits and macros are specifically designed to help programmers transform raw data into submission-ready formats.
In contrast, R and Python lack native support for CDISC models, requiring manual efforts or third-party packages that often lack validation. This is one reason why top-tier Clinical SAS training programs emphasize hands-on experience with SDTM and ADaM conversion. Explore such training methodologies here.
3. Compliance with 21 CFR Part 11
Tools used in clinical trials must comply with 21 CFR Part 11, which governs electronic records and signatures. SAS provides:
-
Comprehensive audit trails
-
Secure user authentication
-
Version control mechanisms
While it's technically possible to achieve this with R or Python, it involves complex setups and custom frameworks—making SAS the safer, faster, and more cost-effective choice for regulated environments.
4. Purpose-Built for Clinical Trial Data
Clinical data is not your typical data. It’s longitudinal, multicentric, and subject to real-time updates. SAS is built to handle this complexity with precision. Its data step programming, PROCs, and macro language make it ideal for managing, transforming, and analyzing trial datasets.
This makes it a crucial skill set for anyone looking to work in clinical research. Find out how clinical SAS skills are used in real-world pharma projects.
5. Widespread Industry Adoption
Global leaders like IQVIA, Covance, Pfizer, and Novartis rely heavily on SAS for clinical data analysis. Their entire ecosystems—from data capture to final submission—are often built around SAS.
This means that most job openings in the domain list Base SAS, Advanced SAS, and CDISC as mandatory skills. See what skills are currently in demand in this domain.
6. R and Python: Powerful, But Not Submission-Ready
R and Python excel in data visualization, machine learning, and statistical modeling. However, when it comes to regulatory submission, they fall short on validation, compliance, and reproducibility. They're excellent for early-phase exploratory research, but not for the final leg of drug approval.
That's why many professionals choose to learn SAS first, and then complement it with R or Python based on project needs. Here’s a smart path to follow.
SAS continues to be the industry benchmark for regulatory submissions because it delivers what regulators and sponsors need most: precision, traceability, and trust. For those looking to enter the clinical research industry, acquiring strong SAS skills remains one of the most strategic moves to build a future-proof career.